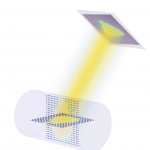
Mission
We study and advance the foundations of data-driven science and engineering.
We power scientific discovery, driven by AI and statistical science.
We are a group hosted at the Division of Scientific Computing, Science for Life Laboratory (SciLifeLab).
We work on the fundamentals of machine learning and AI, with a strong focus on solving hard problems in (life) sciences.
Funding agencies enabling our research:
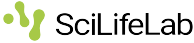
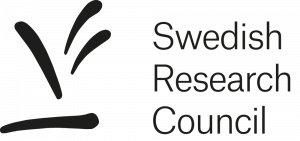
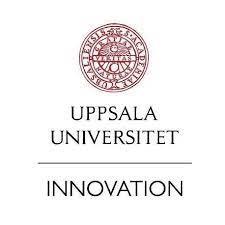
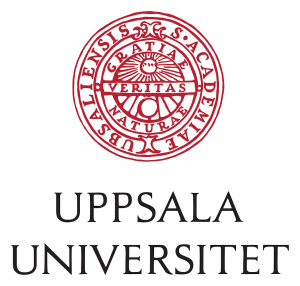
Recent News
– July 23-25, 2024: Aleksandr Karakulev will present our take on parameter-free robust learning via variational inference at ICML 2024 – arXiv link.
– June 05, 2024: Csongor Horváth defends his thesis on studying solution quality for ill-posed optimization problems.
– Nov 07, 2023: Swedish Research Council (VR) starting grant awarded to Prashant Singh.
– Oct 23, 2023: We welcome Andrey Shternshis as a PostDoc in our lab.
Spotlight: Jan 2024
Recent student projects:
– Bayesian Sequential Model Optimization for Drug Combination Repurposing (Dhanushki Mapitigama, Mina Badri, Ema Duljkovic)
– Bayesian Optimization for Characterising Quantum Entanglement (Stefanos Tsampanakis, Ramin Modaresi, Niklas Kostrzewa)
– Deep Learning for Ill-Posed Inverse Problems in Photonics (Johan Rensfeldt, Fredrik Gillgren, Gustav Fredrikson)
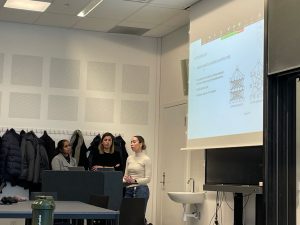
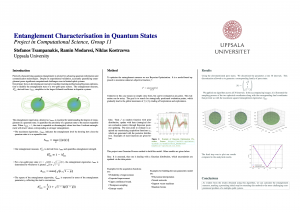
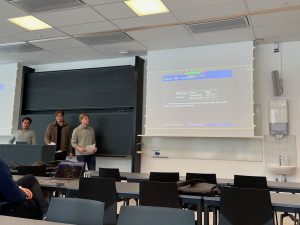
Research Areas
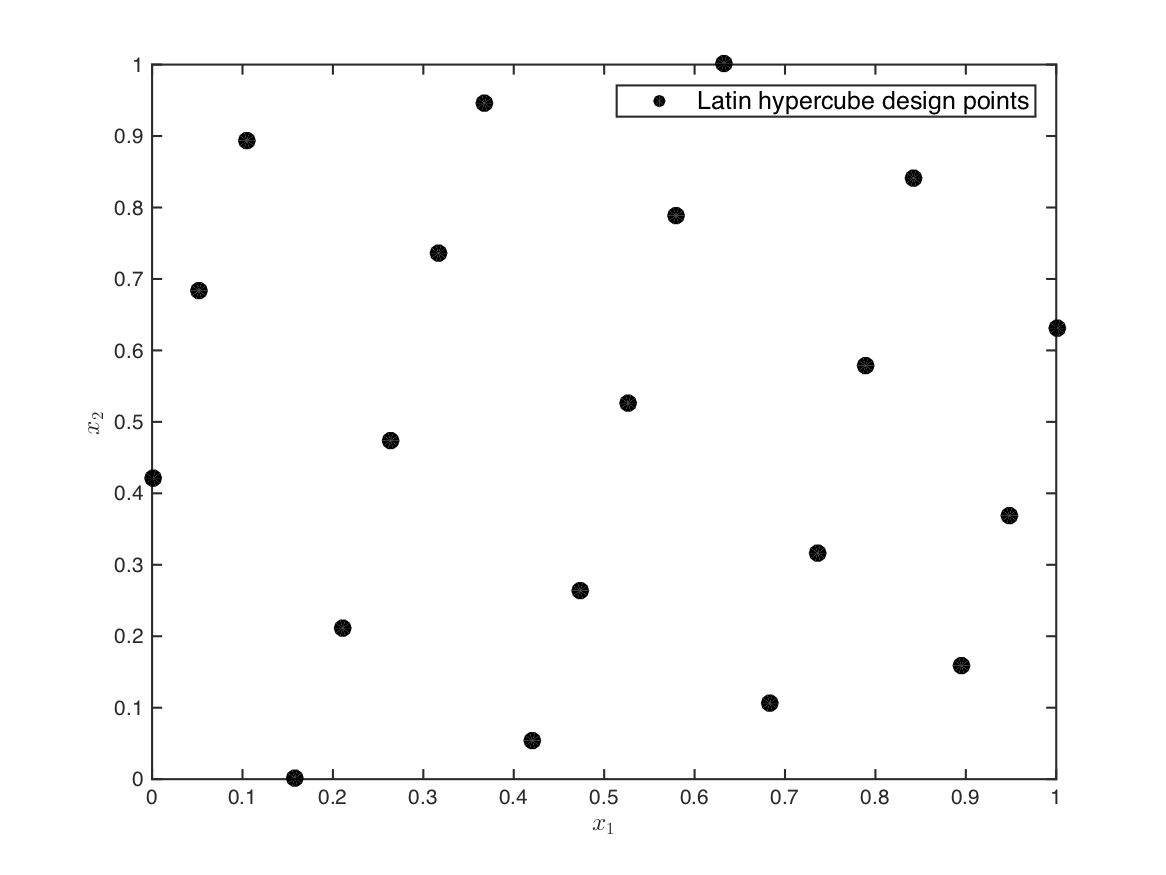
Sampling
statistical sampling, data gathering, active learning, design of experiments, sequential design, reinforcement learning
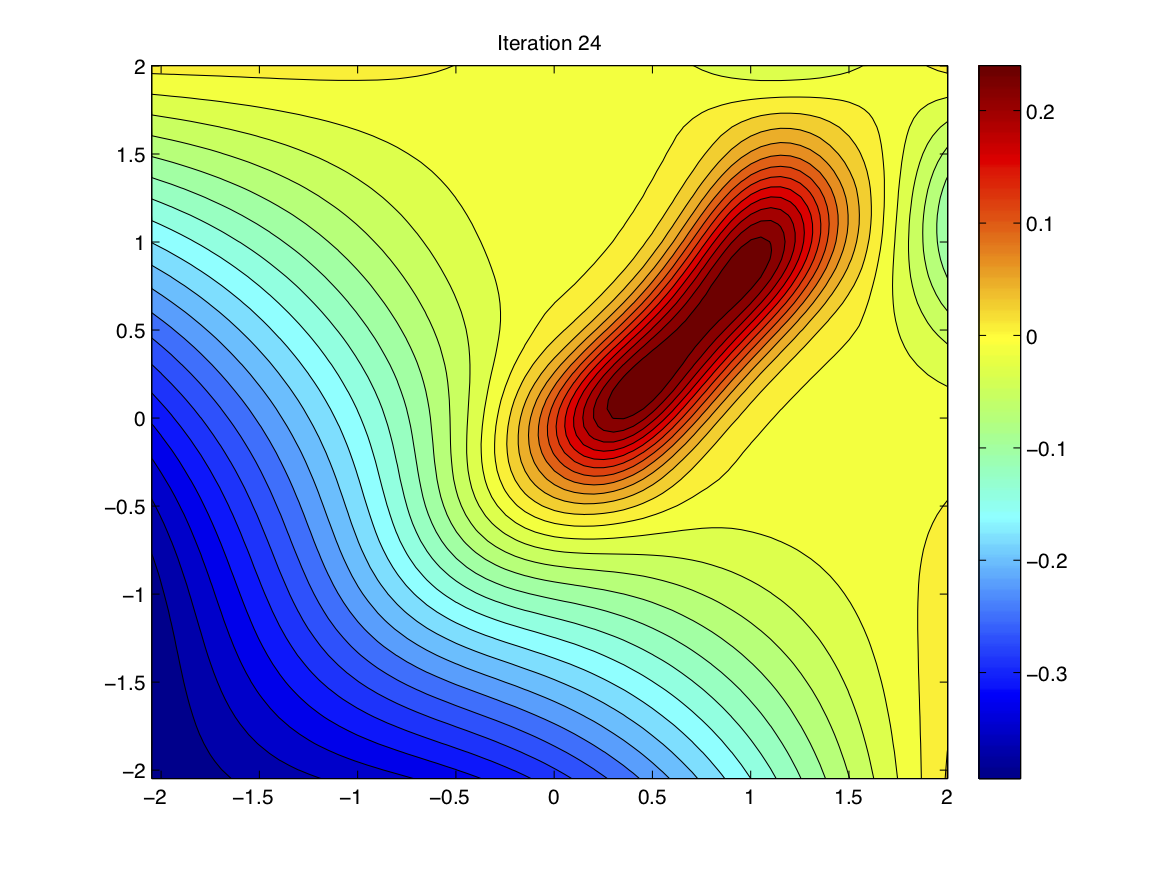
Modeling
surrogate modeling, multi-fidelity modeling, deep learning, Bayesian models, time series modeling
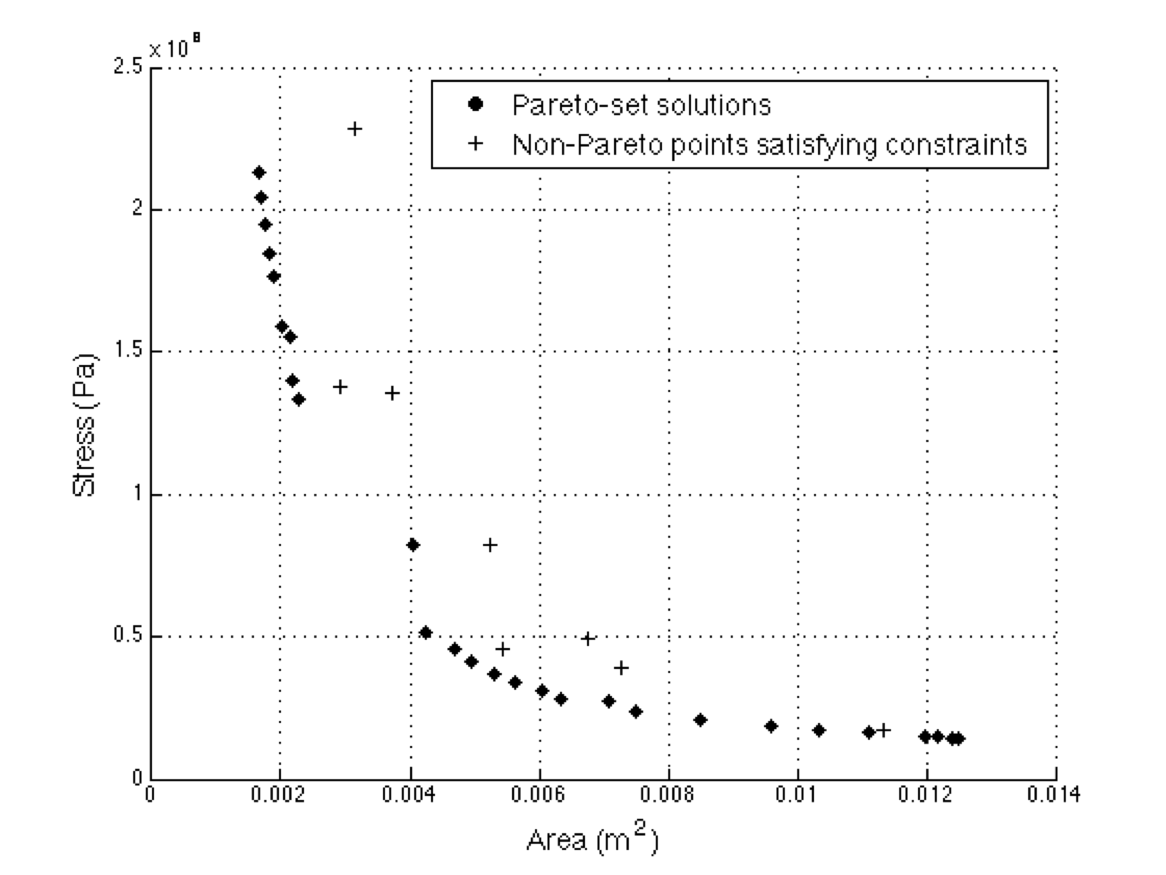
Optimization
Bayesian optimization, non-convex optimization, variational inference, surrogate-based single and multi-objective optimization
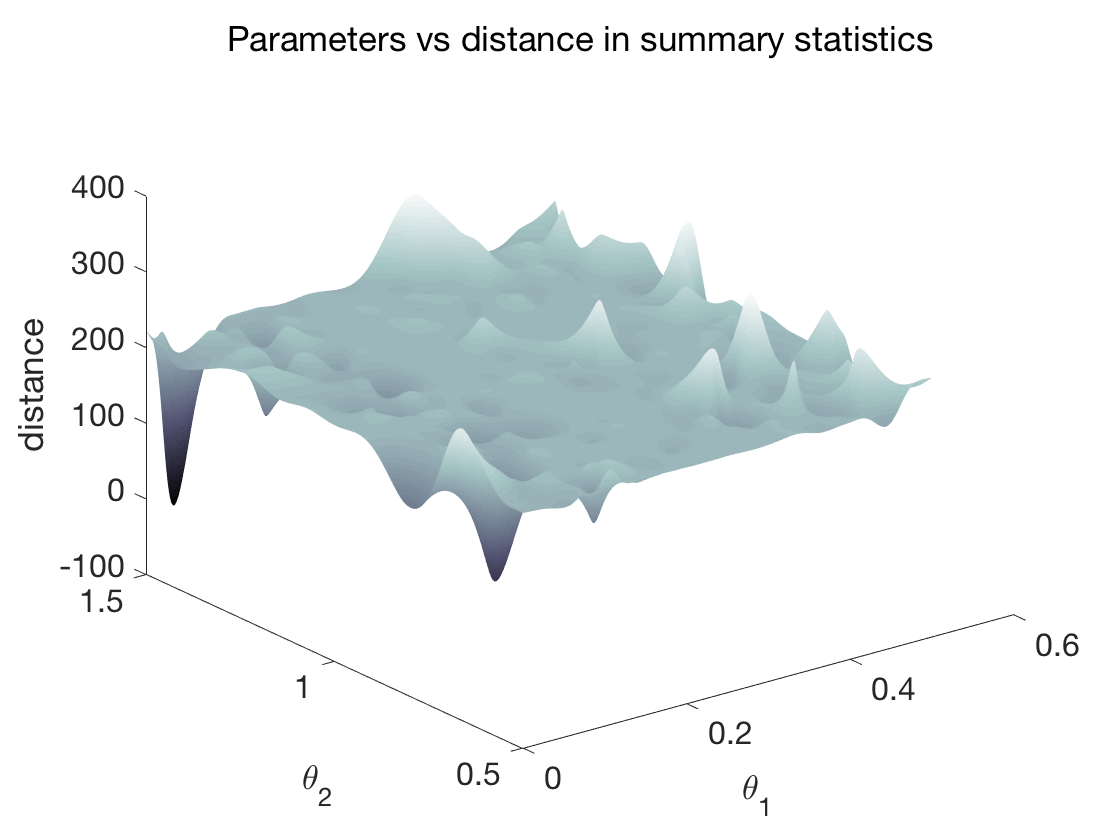
Inverse Problems
likelihood-free parameter estimation, approximate Bayesian computation, learning summary statistics, ill-posed problems